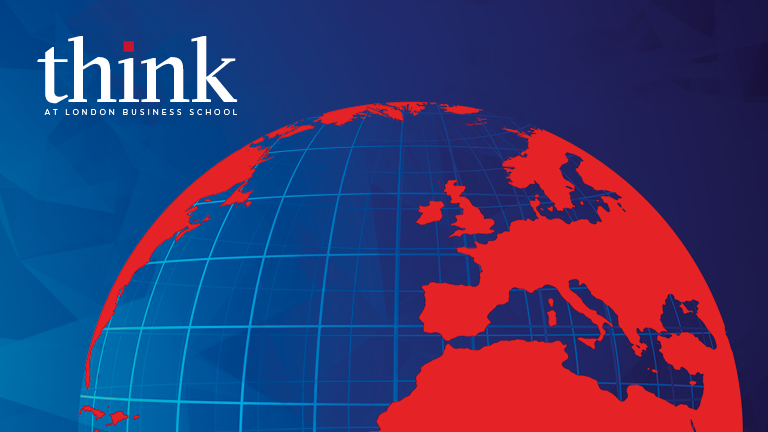
Discover fresh perspectives and research insights from LBS
Think at London Business School: fresh ideas and opinions from LBS faculty and other experts direct to your inbox
Sign upPlease enter a keyword and click the arrow to search the site
Or explore one of the areas below
By leveraging the potential of artificial intelligence, leaders can manage both strategic risks and undesirable risks such as cyberattack
Think at London Business School: fresh ideas and opinions from LBS faculty and other experts direct to your inbox
Sign up