From Behavioural Insights to Business Growth Build your brand through customers insights to drive profits with our short course.
Seeing the future
Is forecasting folly? Lucrezia Reichlin weighs up the arguments
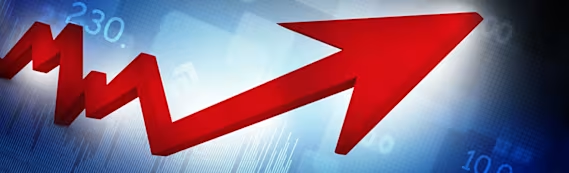
Please enter a keyword and click the arrow to search the site
Or explore one of the areas below
Is forecasting folly? Lucrezia Reichlin weighs up the arguments
From Behavioural Insights to Business Growth Build your brand through customers insights to drive profits with our short course.