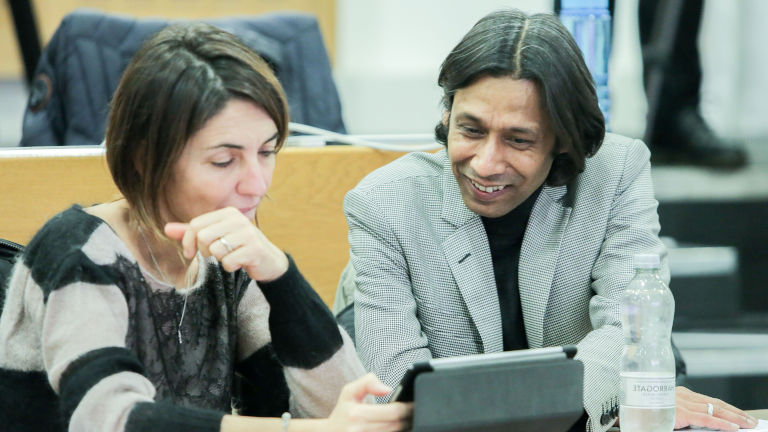
Innovation through Design Thinking
Ignite and sustain innovation into your company's DNA and navigate disruption with our online course.
Read morePlease enter a keyword and click the arrow to search the site
Or explore one of the areas below
Exposing the inner workings of machine learning allows us to take full advantage of artificial intelligence
Ignite and sustain innovation into your company's DNA and navigate disruption with our online course.
Read moreThink at London Business School: fresh ideas and opinions from LBS faculty and other experts direct to your inbox
Sign up